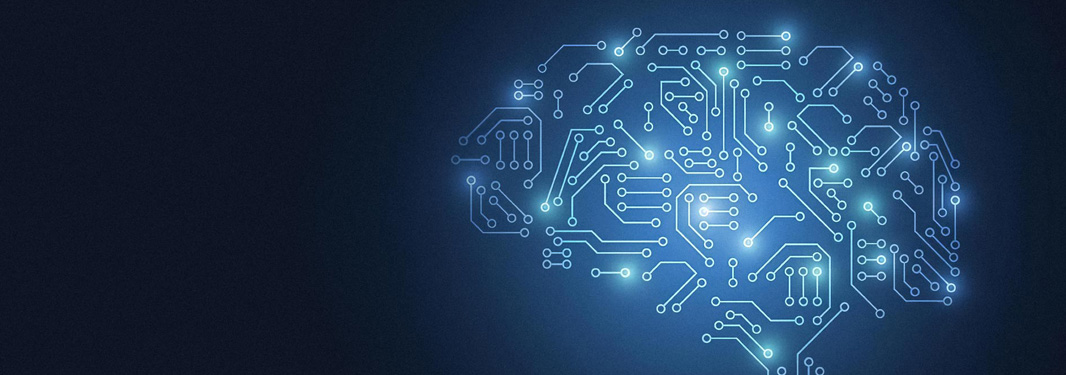
NVIDIA has long been associated with high-performance GPUs, originally designed to accelerate graphics rendering. However, in recent years, these GPUs have become the backbone of Artificial Intelligence (AI) model training and inference.
The latest AI breakthrough is the transformer model which is the foundation for large language models (LLMs) trained today. This model is a neural network that can learn context enabling generative AI. ChatGPT is just one of many use cases of GPT-3, a recent LLM, but the AI opportunity for Nvidia is much broader. Already a $100+bn market, AI is expected to grow at a 39% CAGR through 2030.
Nvidia outlined a 1 trillion-dollar TAM over a year ago. What’s changed over the past year is that the transformer model, which was introduced in 2017, has finally gained broader adoption over the past six months, accelerating demand and broadening AI use cases. While AI was already incorporated in the TAM, the speed at which AI adoption has grown is the upside surprise and is resulting in a valuation boost for Nvidia’s stock.
By leveraging its AI expertise, Nvidia has developed a unique portfolio of not only hardware, but also software offerings aimed at democratizing AI, making the company well-positioned to benefit from the adoption of AI workloads.
Our Nvidia thesis is based on 3 pillars: (1) exponential growth in hardware (2) software introductions (3) automotive opportunity.
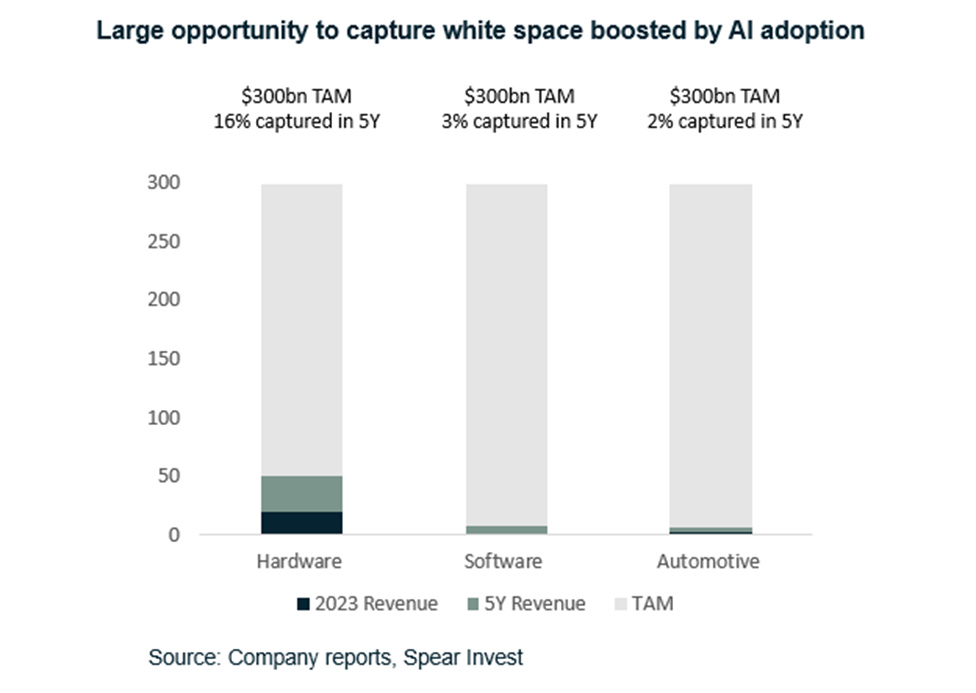
While we expect to see new entrants, it is very difficult to replicate the AI ecosystem that Nvidia built over the past 10 years. Consequently, we expect that the company will be able to capture a meaningful part of the white space. The TAM estimates above were provided by the company and our assessment is that they are directionally correct based on AI industry reports and our channel checks.
Exponential growth in hardware
Over the past 10 years, Nvidia’s Data Center segment has grown from close to 0 (~$200 million in 2013) to >$15 billion today. Moreover, the segment ~doubled over the past 2-3 years implying that we are still in early innings.
The applications for accelerated computing (GPUs) outside of gaming have grown at an exponential rate. Nevertheless, today only 15% of cloud servers are accelerated. We expect that AI adoption will drive this rate >50% as more applications require parallel processing. We expect that GPUs will become the dominant processor, overcoming traditional x86 based server CPUs.
Today, Nvidia commands >80% market share in AI processing units used in the cloud and in data centers.
CUDA architecture provides key competitive moat. The driver behind the high market share is Nvidia’s CUDA architecture (Compute Unified Device Architecture) which is a full development architecture for doing computations on GPUs. CUDA comes with many frameworks and libraries that developers can use for different applications across many industries for free. CUDA communicates down to the GPU and can be used exclusively with Nvidia’s hardware. This is the reason behind Nvidia’s GPUs being the AI standard.
Growth in model sizes requires advanced hardware. AI model sizes are growing at an exponential rate and AI workloads are only at the cusp of their potential. Increased number of parameters means exponentially higher number of GPUs and DPUs, which is the core competency of NVIDIA. Any model with more than 1.3 billion parameters cannot fit into a single GPU. Models have grown from <100million parameters 2-3 years ago to 100s of billions today.
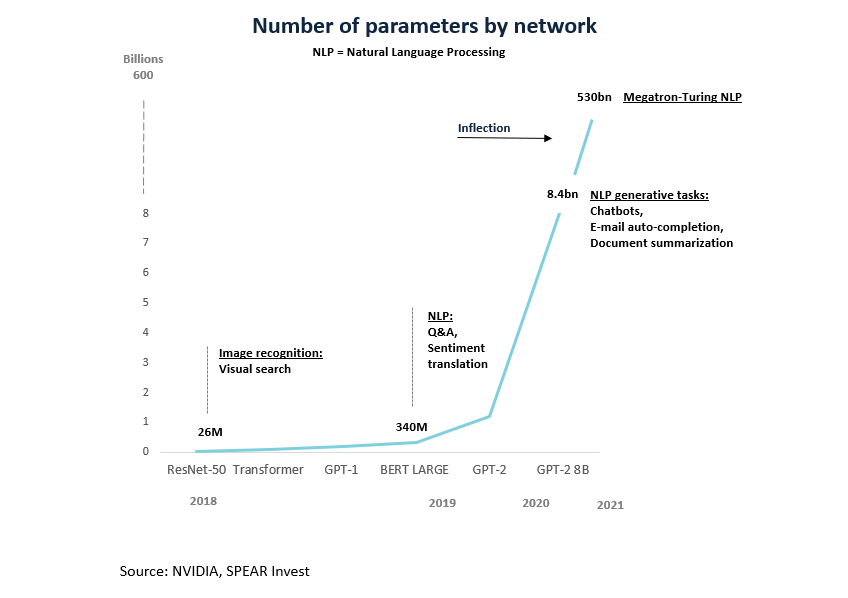
Data center hardware comes with a high price tag. Nvidia’s data center products are customized for AI applications and are sold at significant premiums. Data Center products are significantly more profitable than gaming and are the driver behind Nvidia’s gross margin increase from ~40%s in 2010 to >55% today. As a reference point, the price for data center graphic cards is $20-30K vs. gaming cards $2-3K. Moreover, new product introductions such as Hopper 100 (H100) replacing the popular Ampere (A100) carry 2-3x higher prices. Per the company, H100 runs 30x faster than its predecessor when deploying models and requires 6 to 9x less infrastructure to train models.
Software valuation framework
While the CUDA architecture is free to use, over the past 2 years, Nvidia introduced two software offerings (1) Enterprise AI and (2) Omniverse, which could become meaningful growth drivers over time.
Today software represents only $400-500 million in revenues and is at a similar point as hardware was 10 years ago. Using what we believe are conservative assumptions we arrive at ~$7bn+ software opportunity in the next 5 years growing at double digits going forward, which we value at $80-100bn.
Below we outline the potential for each product:
Enterprise AI is a software suite comprised of solvers and libraries that NVIDIA has developed over the years aimed at putting accelerated computing in the hands of every company. This product is part of the Data Center segment. The company’s partnership with VMware vSphere significantly increases scalability and ease of implementation as 85-90% of IT today runs on VMware. This suite includes the horizontal layers of Nvidia software stack and will be sold along with vSphere in a standard software license model. It is priced at $3,600 per CPU socket and gives customer access to NVIDIA middleware layer (CUDA-X).
Applications specific vertical layers are offered for free. They include Metropolis for smart cities, Clara for AI powered healthcare, Riva for interactive conversational AI, Merlin for AI recommenders, Maxine for video conferencing, Isaac for robotics intelligence, and Morpheus for cybersecurity. Early adopters of these frameworks include Pinterest, Spotify, GE Healthcare, T-Mobile etc.
In addition to purchasing Enterprise as a software suite, Nvidia introduced a full offering which includes both software and hardware (DGX Superpod), where NVIDIA manages the infrastructure and customers pay on a monthly basis. The goal of this offering is to reduce the barriers to entry for smaller customers. Base Command (platform for training and developing AI models) and Fleet Command (product that enables companies to deploy and manage the AI models out to the edge) both part of NVIDIA’ AI LaunchPad hybrid-cloud partner program.
NVIDIA provided a framework for estimating the market opportunity for Enterprise AI. The vertical market and the infrastructure-as-a-service offerings (Base Command and Fleet Command) are harder to estimate but we believe they could offer equally large opportunity.
Moreover, on the software side, it was assumed that Nvidia would originally target enterprises, with hyperscalers potentially developing in-house solutions. But recently Nvidia announced several partnership with the hyperscalers where AI is offered as a supercomputing service.
(millions) | |
Server units shipped annually | 12 |
Sockets per server | 2 |
Total sockets | 24 |
Enterprise share (excluding hyperscale) | 40% |
NVIDIA’s addressable market | 9.6 |
Market share | 30% |
Sockets with NVDA AI | 2.88 |
Revenue per socket* | $1,260 |
Revenue per year | $3,629 |
For revenue per socket, we use $900 annual maintenance
+ $3,600 per CPU socket amortized over 10 years
Source: SPEAR Invest, Nvidia
Omniverse is the other software offering which is part of the Pro-Viz segment. It is a collaboration platform made to create virtual scenarios and can be used to simulate cities, factories, airports, robotics, engineering projects, etc. Current users of Omniverse are: BMW using it to achieve 30% more efficient throughput in factories, Bentley using it for designing and real-time testing of infrastructure projects and many others.
Key with Omniverse is that it is a platform with open standards, cloud native, multi-GPU scalable, and connects a large ecosystem of applications and software such as Adobe, Autodesk, Epic Games, Blender.
The offering is priced on a subscription basis with 3 components: Nucleus, Creator, and Viewer. Nucleus is prices on per CPU socket ($5k) and each individual Creator license is $1,800 per user. NVIDIA believes that there are ~30mm creators (engineers, designers etc), so assuming a single digit adoption rate by 2026 implies a multi-billion-dollar opportunity in the next 5-10 years.
(millions) | 2026E | 2030E |
Potential users | 20 | 20 |
Market share | 8% | 15% |
NVIDIA Omniverse customers | 1.6 | 3 |
Per user subscription | $1,800 | $1,800 |
Revenue contribution | $2,880 | $5,400 |
Source: SPEAR Invest, NVIDIA
Enterprise fee for Nucleus incremental to this analysis
There are three important things to take away: 1. Omniverse/Enterprise AI can both be multi-billion dollar businesses 2. Given the early innings, NVIDIA could realize double-digit topline growth for 15+ years 3. Software revenues come at a high margin, and therefore significantly higher earnings multiple.
Automotive Opportunity
In addition to AI hardware and software, we expect that Nvidia’s core technology will serve as the backbone for autonomous driving. Outside of Tesla, which is developing its in-house autonomous driving system, Nvidia is likely to power most other OEM systems.
With 100 million cars sold per year and an installed base of over 1 billion vehicles on the road, we expect this to be a significant opportunity for Nvidia. The company currently has $11bn+ in automotive backlog which it expects to convert over 6 years.
As an example, Mercedes alone expects to generate $2 billion in revenues from car-subscription services by 2025, and high-single- digit-$billions by the end of the decade. Mercedes has a partnership with Nvidia, where profits are split 50/50. As a reference, Nvidia’s Auto segment has only ~$300million quarterly revenue today and Mercedes is just one of the customers, implying a huge runway.
Nvidia has also gained significant traction with Chinese OEMs. Currently, these companies use Nvidia’s hardware (namely DRIVE Orin SoC) and are developing their own software, but we have noted interest in leveraging Nvidia’s full platform from several players. This relationship can be a win-win as it enables OEMs to innovate faster and could establish Nvidia as a dominant AV platform.
While the revenue (and backlog) today is derived mostly from hardware, similar to the Data Center segment, we expect that over time the opportunity will extend from hardware to software and model access/training. Below is an overview of Nvidia’s automotive products and datapoints on adoption:
- Vehicle Hardware (SoC): Nvidia’s DRIVE Orin is currently used by ~25 (out of 30 major) vehicle manufacturers including all major Chinese EV producers (NIO, Li Auto, XPeng, BYD etc). EV OEMs are rolling out vehicles ranging from a single Orin SoC (254TOPs — trillion operations per second) to up to 4x Orin SoCs. We estimate the content per vehicle to be ~$300. At 50% penetration implies $15bn annual opportunity (from <$200mm in 2021)
- Vehicle Software + Hardware: Hyperion, an end-to-end platform connecting cameras, sensors, radars, and LiDAR. Mercedes and Jaguar were the early adaptors with model introductions coming in 2024. Hyperion is open and can accelerate the AV time to market by giving manufacturers the ability to leverage Nvidia’s own development work and providing ongoing upgrades (e.g., Hyperion 9 is expected to come in 2026 and feature 14 cameras, 9 radars, one lidar and 12 ultrasonic sensors).
- Supercomputer/model training: While Drive Orin serves as the brain and Hyperion as the nervous system inside the car, model training occurs outside of the car and is yet another opportunity for Nvidia. Most manufacturers are building their own data centers using Nvidia’s hardware (e.g. NIO is using Nvidia’s HGX with eight A100 Tensor Core GPUs). But in the future, Nvidia will be able to enhance its customers’ capabilities, with the Aos supercomputer which the company plans to leverage for model training. Aos is expected to be >4x faster than the world’s fastest supercomputer and 4x faster than Nvidia’s current Selene supercomputer. It is expected to be able to scale to 18.4 Exaflops of AI computing (32 petaflops per DGX system) and consists of 4,608 next-gen Hopper H100 GPUs (8x H100 GPUs per DGX; 576x DGX systems or 18 DGX Super PODS).
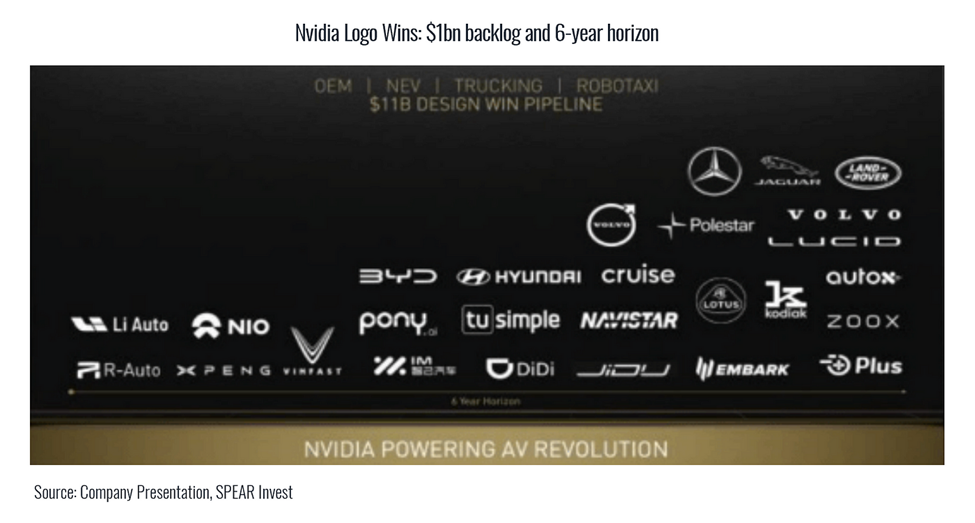
Assumptions and risks
The key input for Nvidia’s valuation is top-line growth and sustainability of that growth. The hardware opportunity offers near-term upside; the software and automotive opportunity are expected to drive the next leg of growth after they achieve scale.
While data center hardware has grown from ~$300million to >$15bn over the past 10 years, the software and automotive opportunities are starting from a small base. We estimate that software represents only $400-500 million today (excluding the existing Pro-Viz business). Similarly, the Automotive segment represents <$2bn today consisting mostly of hardware solutions, but could become a growth driver and margin accretive once it reaches scale.
The key aspect to consider when valuing Nvidia’s stock, is that while for most hyper-growth companies we assume that growth falls off after Year 5, Nvidia’s may just be getting started.
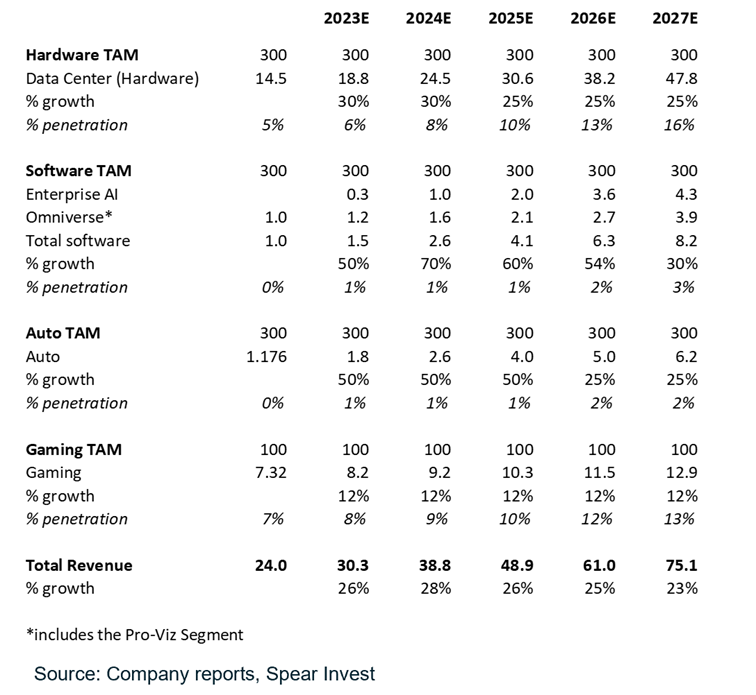
Many investors look at 1-2 year forward multiples, which provide data-points, but not a valuation framework. Most analysts project that the next twelve months will be similar to 2022 (topline and EBITDA), while the demand environment (interest in AI) and the company’s products (e.g., H100) have significantly improved.
Compared to when we first published this report in 2022, the main assumption change in our model is that demand is pulled forward, especially on the hardware side. The ultimate opportunity set has not changed, but contrary to what most investors believe for growth assets, the first 5 years are key for valuations.
We believe that the hardware story is known, but the magnitude of the growth could surprise to the upside. The software and automotive opportunities are lesser known, but if those opportunities materialize, they could drive growth for Nvidia post year 5, roughly the timeframe when we expect to reach scale.
Risks
- It is important for investors to keep in mind that the hardware business is constrained by capacity, silicone availability etc. While it is possible for Nvidia to surprise to the upside on topline driven by pricing, there is a physical limitation to production (at least in the near future).
- Software businesses are sold differently than hardware and may require more effort than initially anticipated. Software carries significantly higher margins which offsets some of this risk.
STAY INFORMED WITH SPEAR
SPEAR publishes original research about industrials and industrial technology.
To subscribe please fill-in the info below: